注:由于已经学过另一门《机器学习》,这里的笔记会比较简略,点到为止。
《机器学习》笔记传送门:Here。
References
完结撒花!吴恩达DeepLearning.ai《深度学习》课程笔记目录总集
Notes from Coursera Deep Learning courses by Andrew Ng-TessFerrandez,PDF
Convex Optimization-Boyd&Vandenberghe
Neural Networks and Deep Learning
神经网络、深度学习
深度学习
最简单的神经网络:修正线性单元ReLU。【一个神经元】
(如图)左侧是数据可视化,右侧是对应的神经元模型。
经典的神经网络是全连接的。这意味着,我们并不去手动选择哪个神经元负责什么方面——每一个神经元都会接收到充分、完整的信息,它们将自动地从全局上进行任务分配,逐渐演变出不同特长的识别能力来。
在小规模数据,算法的性能通常取决于特征工程。而大规模数据,大规模的神经网络则取得高性能。
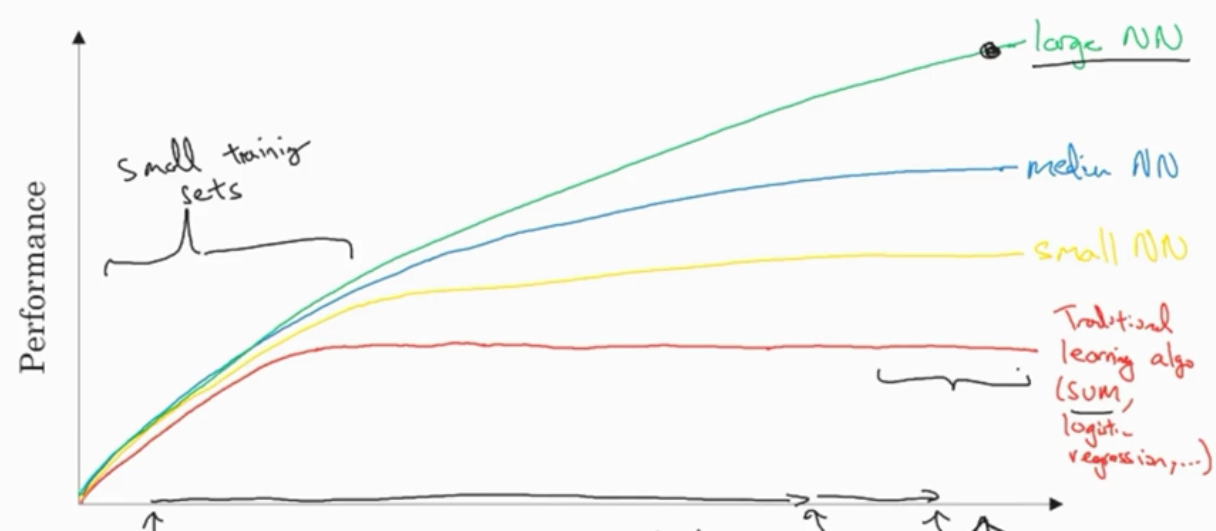
神经网络基础
Cost
函数是Loss
函数的总和。
向量化 vectorization
执行速度快。充分利用并行计算的优势。尽量避免使用显式的for
循环。
广播 broadcasting
广播是函数的向量化(自动复制匹配)。(Here)
Matlab:
bsxfun
技巧 Tricks
用矩阵,不用向量。np.random.randn((5,1))
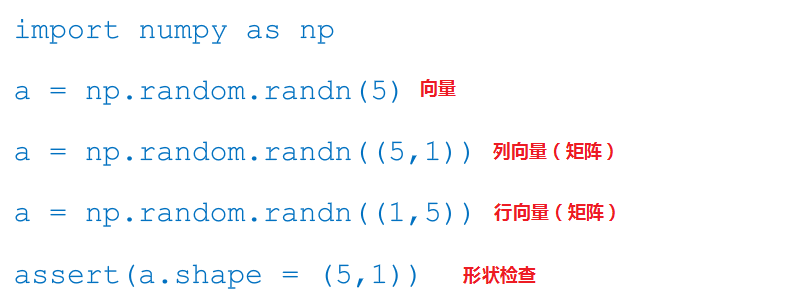
逻辑回归推导
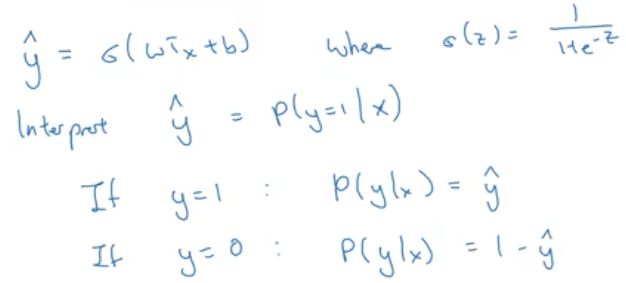
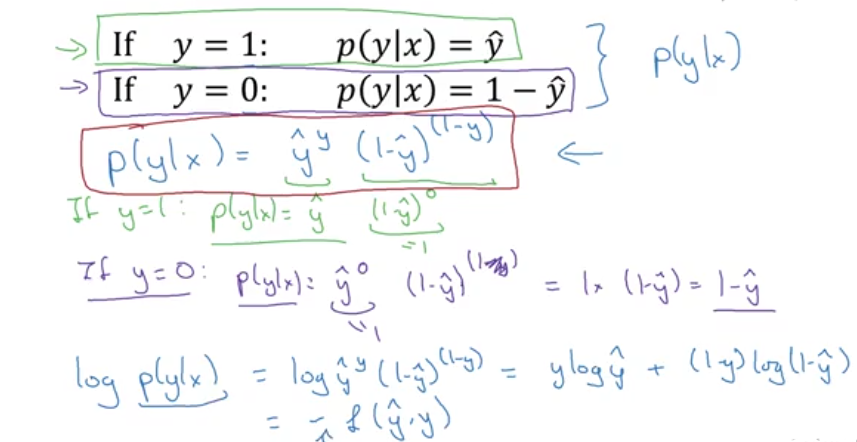
极大似然估计
使给定样本输出的观测值的概率最大化。
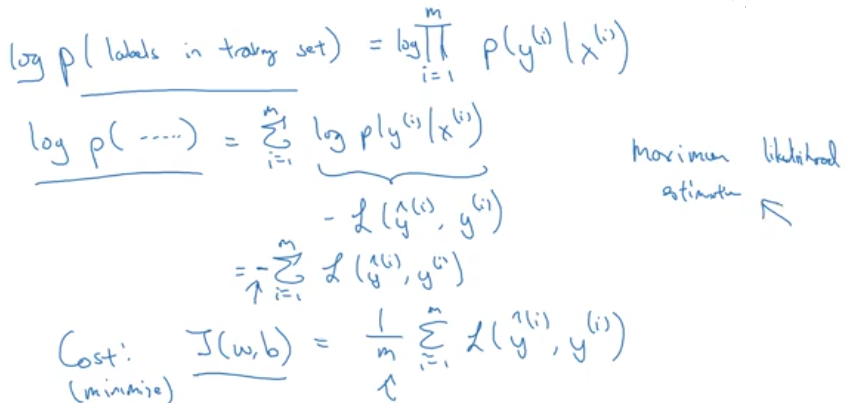
浅层神经网络
非线性的激活函数
一个使用线性激活函数的神经网络层,是没有效果的。不能增加整个神经网络函数的拟合能力。
激活函数梯度
在反向传播时需要计算激活函数的梯度。(作为链式法则的一环)
随机初始化
如果将神经网络的权重设为同一值,那么最后它们总是高度对称的。函数拟合能力因此而下降。
随机初始化为较小的值(0.01左右),保证梯度较大并且保持神经元特异性。
深层神经网络
原理
对于具有层次的问题来说,深层网络能极大节省计算成本。(单层可能需要指数级的神经元)
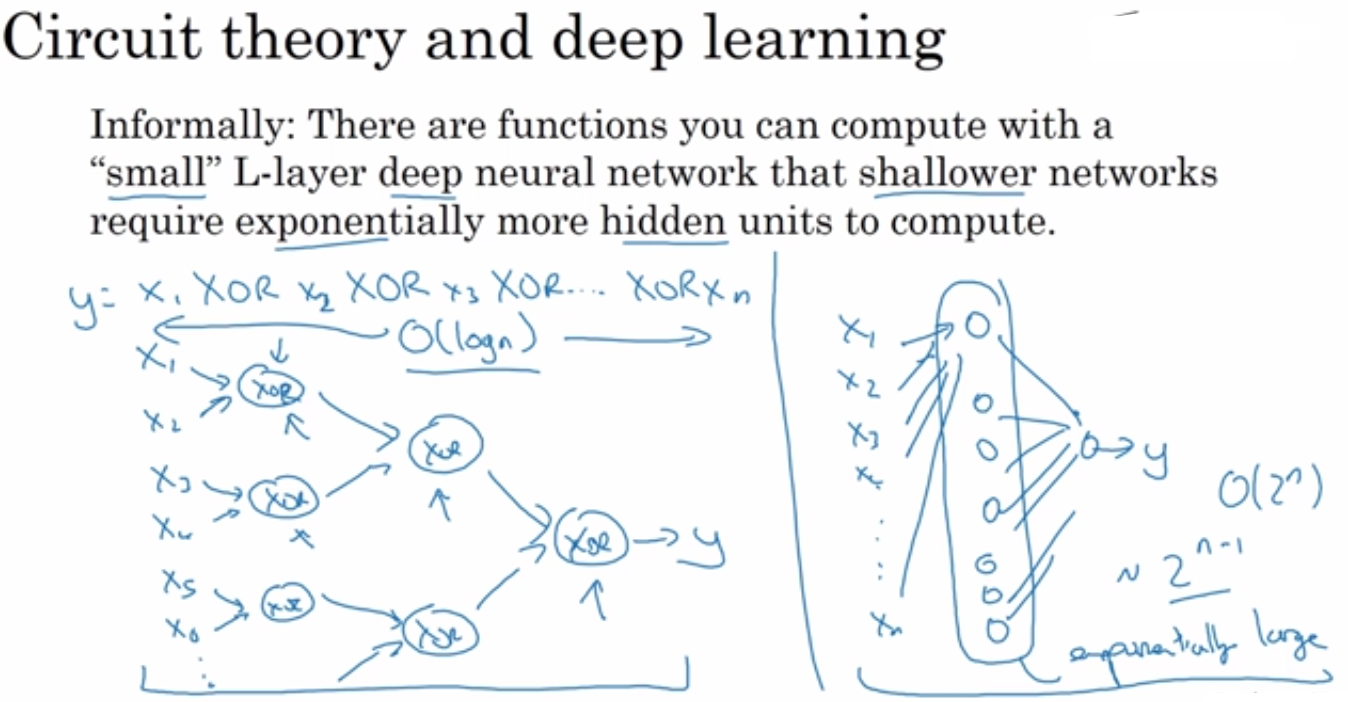
改善-深层神经网络
结构化-机器学习
卷积神经网络
序列模型
循环序列模型
简介
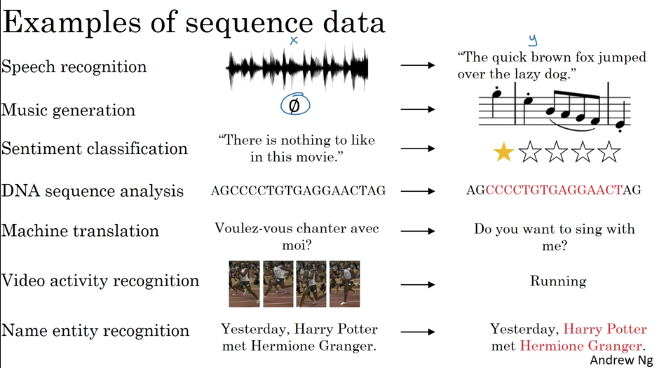
语音识别(Speech recognition)
音乐生成(Music generation)
情感分类
DNA序列分析
机器翻译
视频
命名实体识别
- ……
RNN 循环神经网络
以01标注为例(seq=seq)。(应用:命名实体识别)
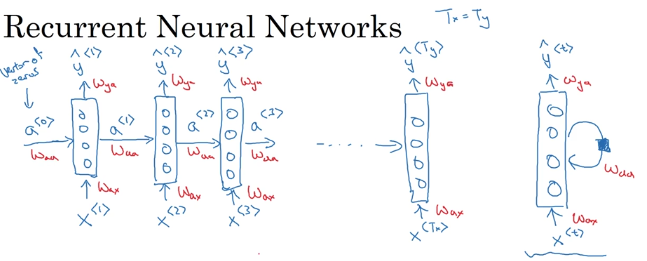
缺点:只利用前缀信息。(但对于时间序列来说则刚好)
穿越时空的传播
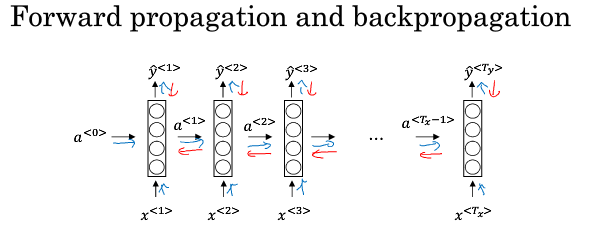
计算图如下:(从右到左的时光倒流)
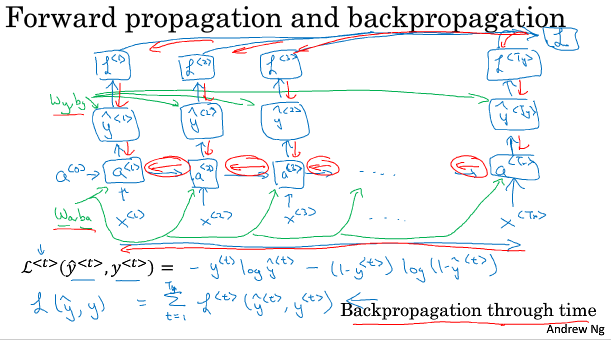
架构
左边是等长序列模型,右边是变长RNN(分为encoder
和decoder
)。
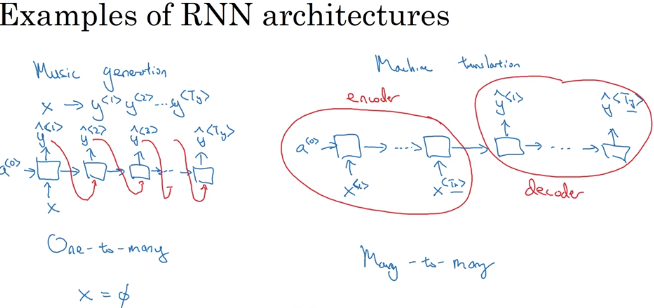
总结
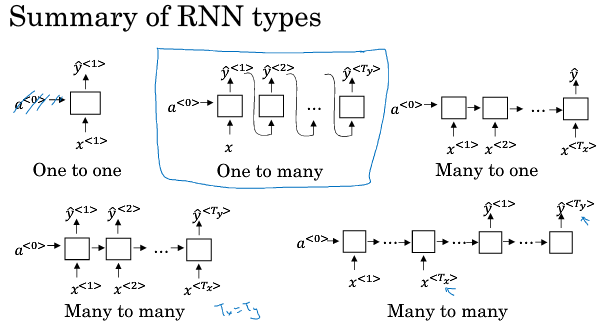
基于字符的语言模型不能很好地捕捉长程关系,训练成本也更高。
传统的RNN局部性较强。长程容易出现梯度消亡、梯度爆炸现象。
GRU
c=memory cell
。
按照激活函数来判别相关性
以及是否继承记忆
。
Simple RNN Unit
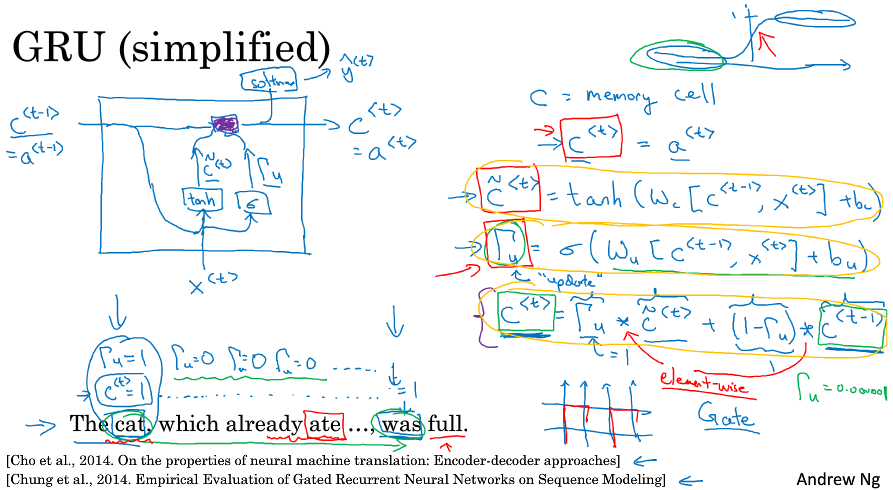
GRU
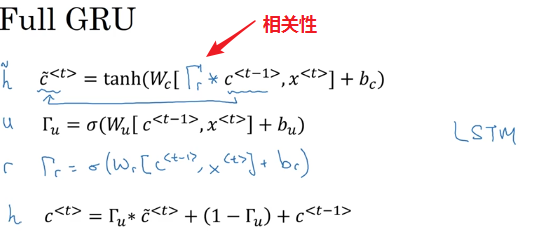
LSTM
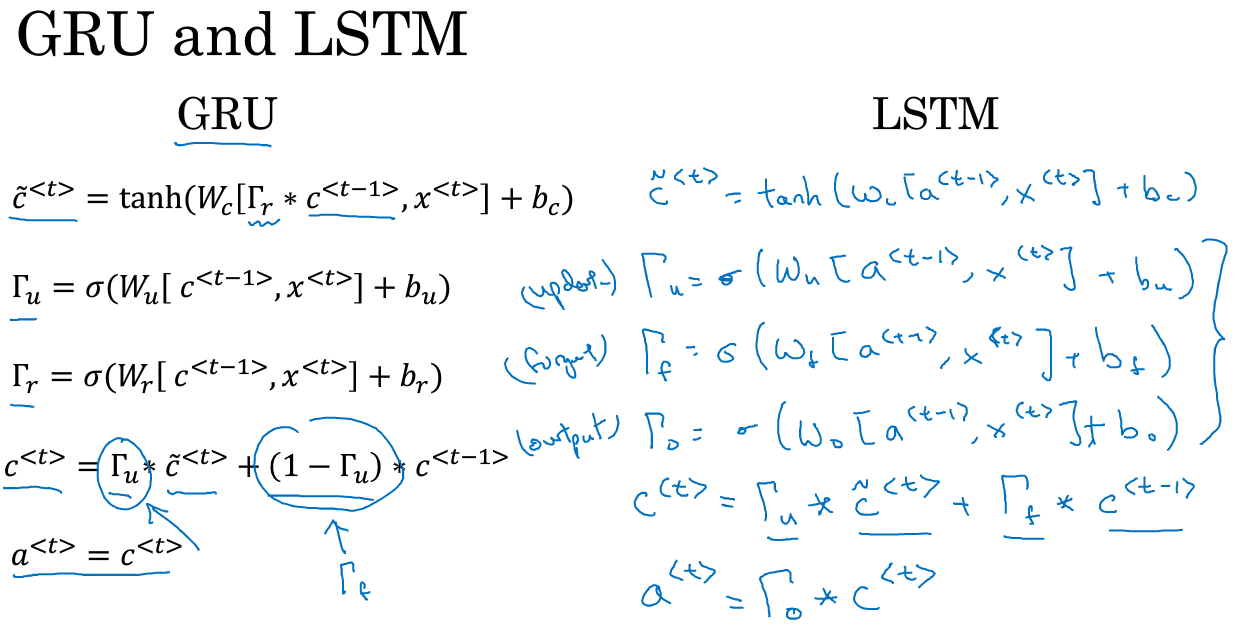
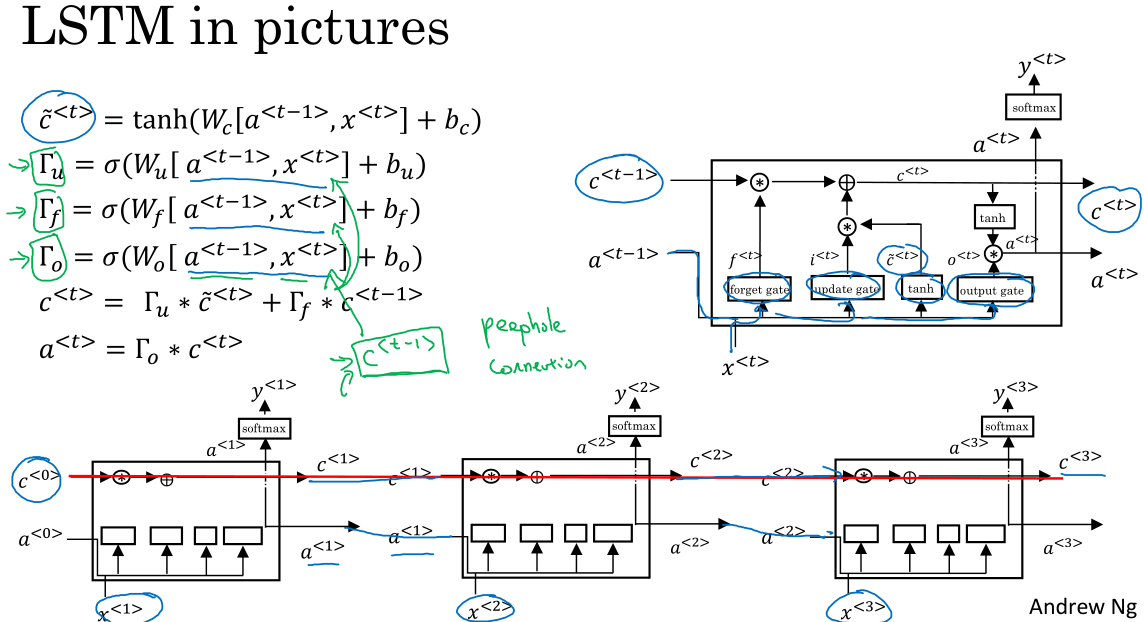
LSTM更强大更通用。
GRU更快更省,更易扩展到大规模数据。
BiRNN
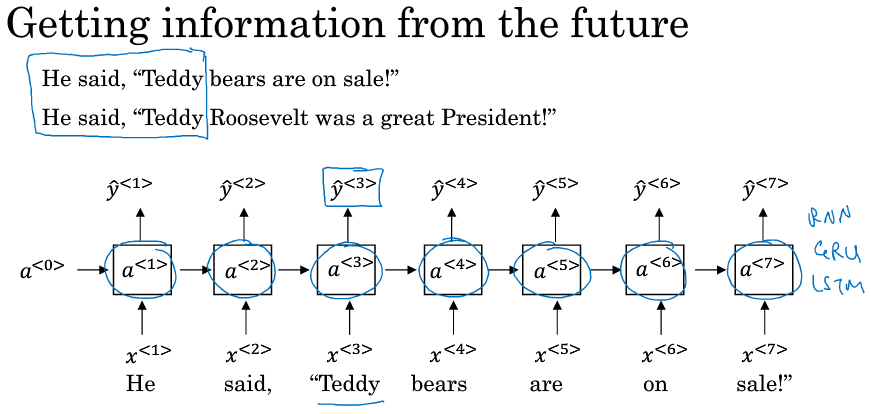
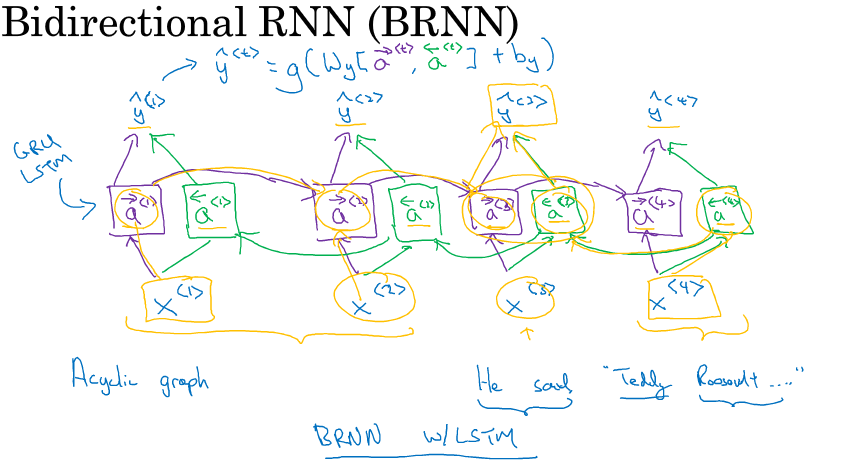
深度RNN
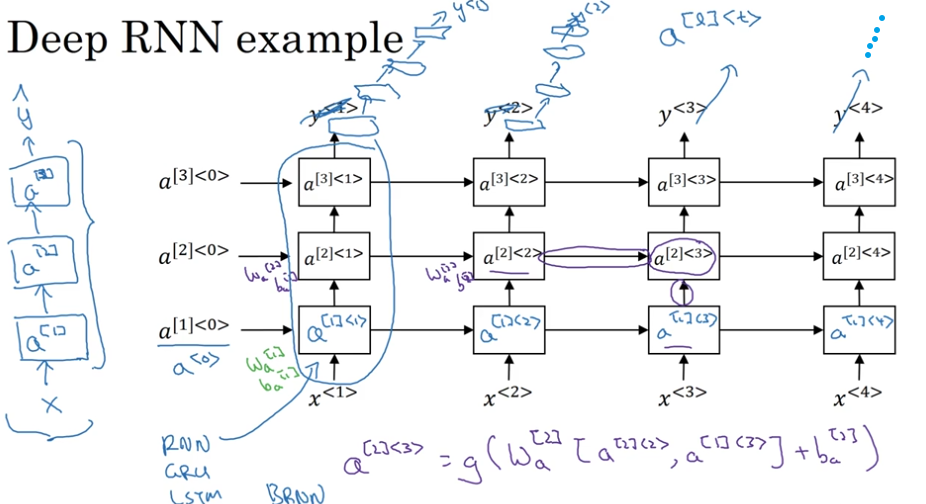
自然语言处理
one-hot representation
: 无法表示相关性。
featurized representation
: 利用人工制造的特征来表示word。
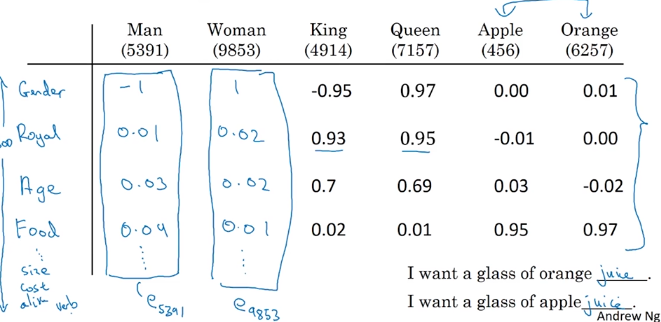
Visualizing and Embedding
t-SNE
可视化算法。
Transfer learning and word embedding
从大规模数据集迁移到小规模数据集。
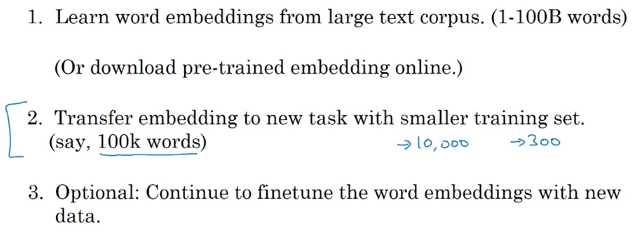
Analogies 推理
联想的相似性:(embedding空间的平行四边形)
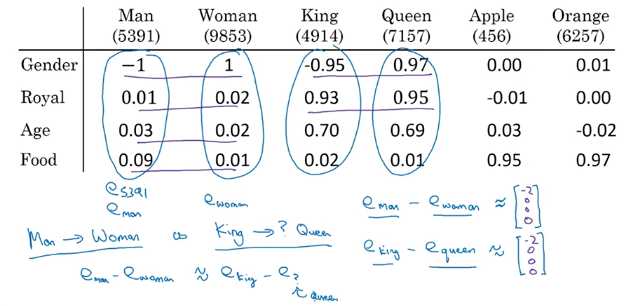
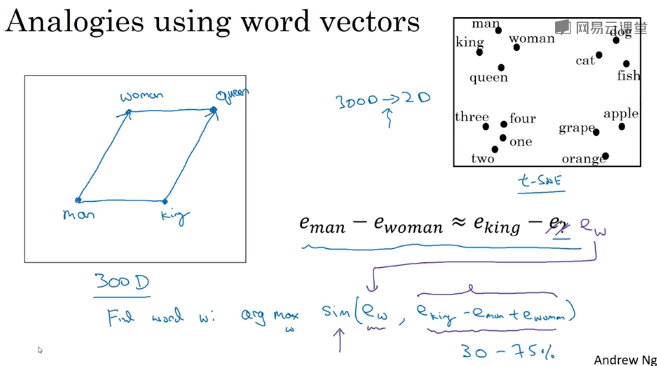
余弦相似度
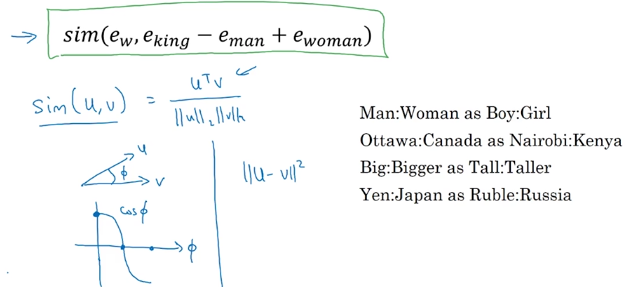
Embedding matrix
keras: embedding layer
.
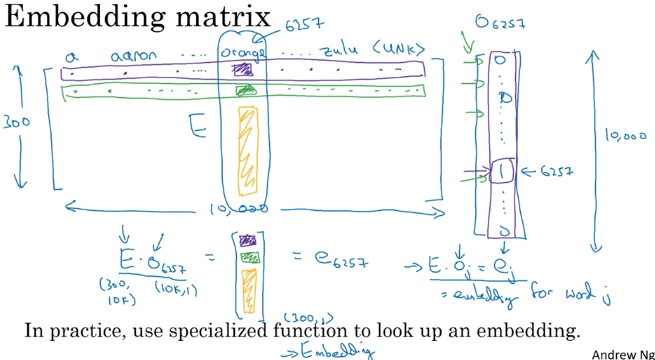
学习词嵌入
自然语言模型
学习一个自然语言模型是一个获得embedding的好方法。
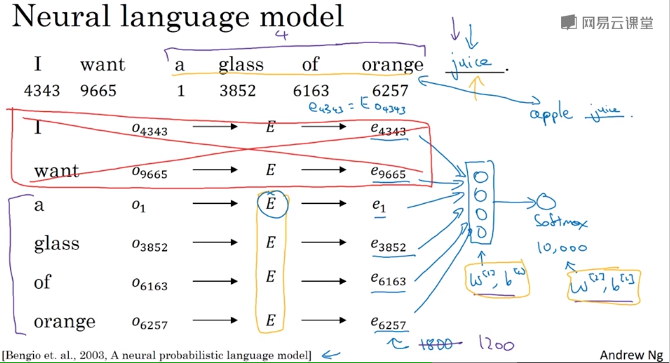
其它上下文相关
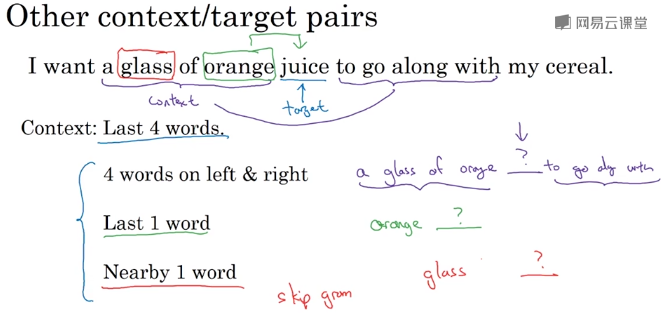
Word2vec
从上下文随机选择。
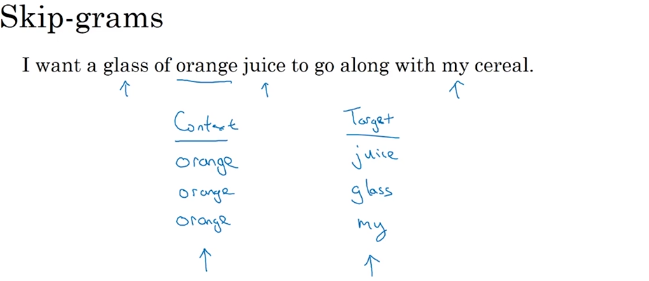
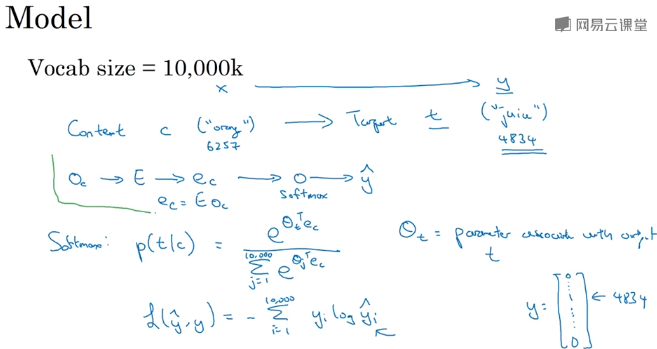
层次softmax
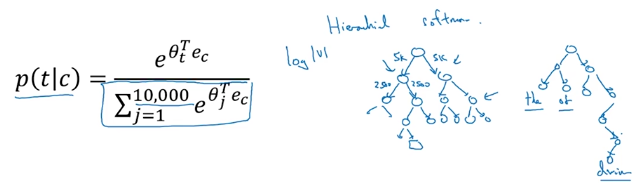
采样时可能还需要平衡词频。
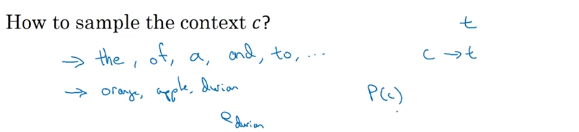
负采样
随机从数据集中选择,那么这些随机选择的单词应该构成负样本。
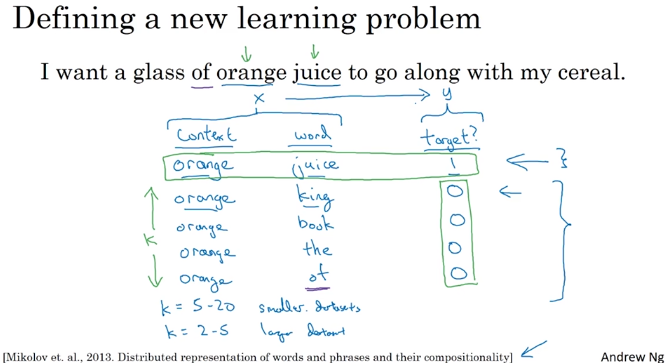
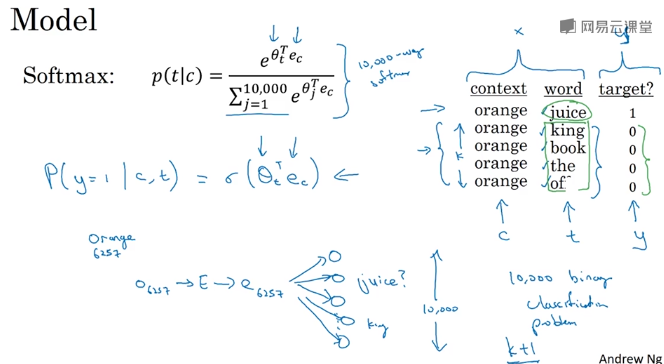
采样经验频率:(${w_i}^{3/4}$)
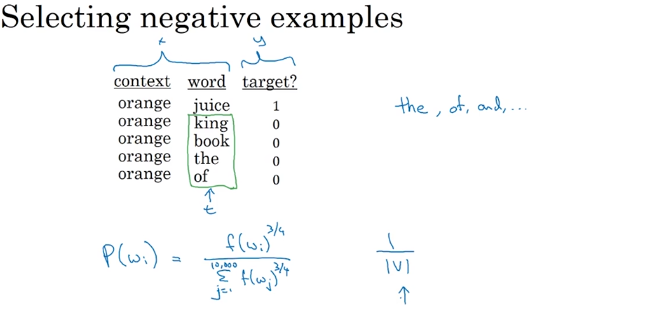
GloVe(Global Vectors for word representation)
情感分类 sentiment classification problem
小样本。
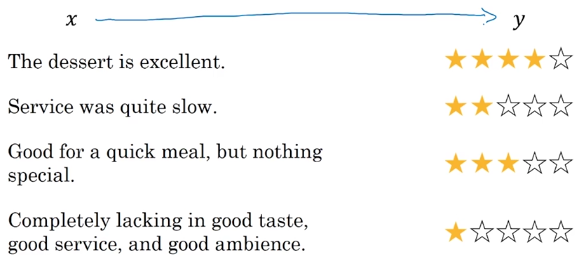
平均法
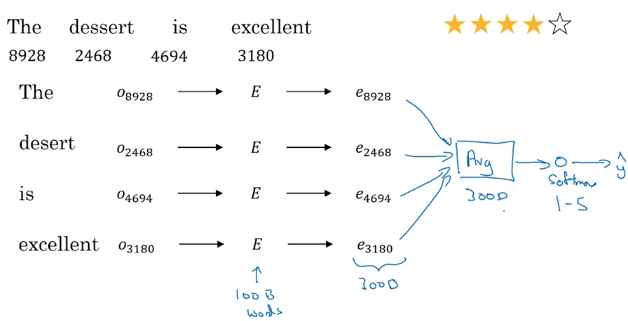
缺点:忽视词序。
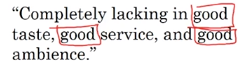
RNN for sentiment classification
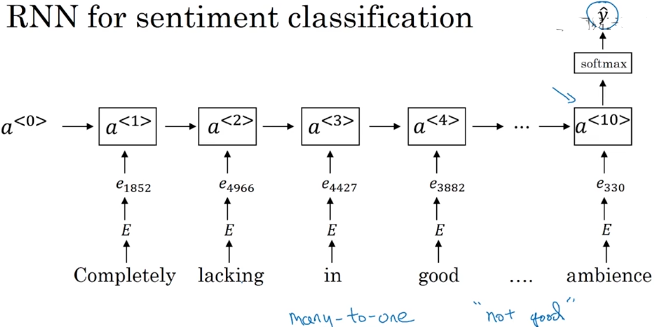
词嵌入除偏
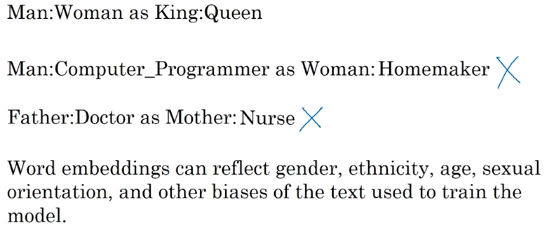
减少AI中的bias更容易也更重要。
- 机器学习学到社会习俗而非自然规律。
移动那些有bias的词,使得它们到两种gender的距离相同。
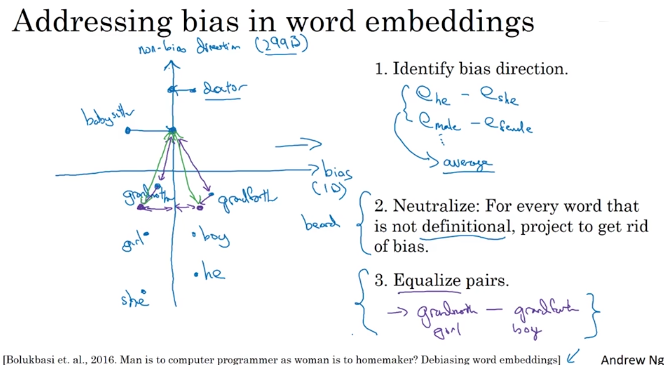
序列模型、注意力机制
基础模型
seq2seq
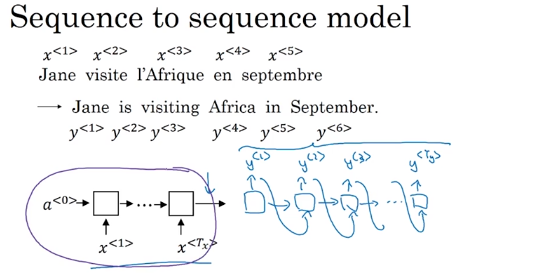
Image captioning
还是RNN的模式。
选择最可能的句子(分离encoder和decoder)
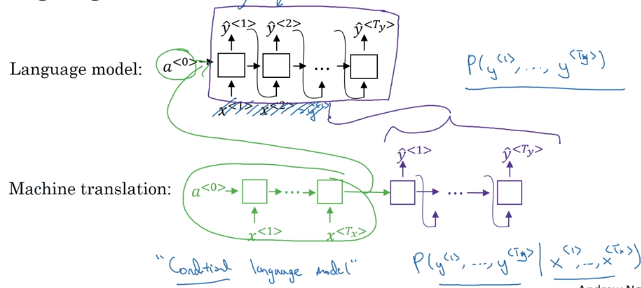
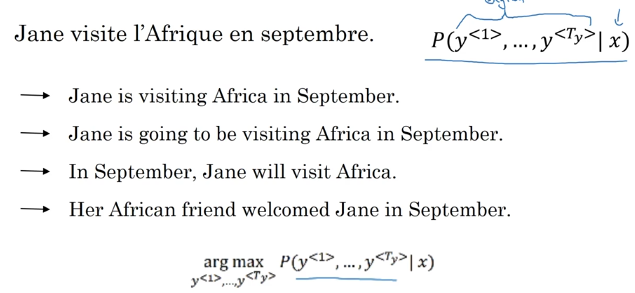
贪心搜索的效果并不好。
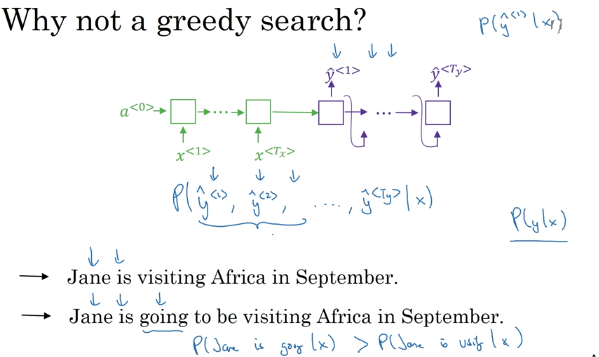
选择一种近似的算法。
定向搜索算法 Beam search
设置一个beam width(比如3),形成一个有限的次优候选词列表(比如最大概率的3个词)。
人工智能采访专辑
Geoffrey Hinton
全息图:分布式记忆。
切去大脑的一半,仍然能获得大脑完整的信息。
符号主义与联结主义之争。
幻想学:符号主义+联结主义。(存在一种虚幻的终极表示,但真实的形态可以任意的)
可解释性是AI得到接受的一个动力。
算力的剧增是AI得到应用的动力。
胶囊网络(capsule):一组神经元称为一个胶囊。
读论文,但也不必读太多。(创新型工作)
永远不要停止编程。
Pieter Abbeel
深度强化学习。
- 数据的收集很难
- 需要负例
- 长时间的稳定性
关注关联性。
真正着手去做!
Ian Goodfellow
深度学习研究员。(GAN
发明者,花书
的作者)
Game AI。
GAN(对抗生成网络):一夜之间灵感诞生。
GitHub
开源项目。在ArXiv发表文章。
对抗样本:机器学习安全。